Generative AI has many biases, human ones in particular. Our Data & AI experts can help you to understand and control the biases found in Generative AI.
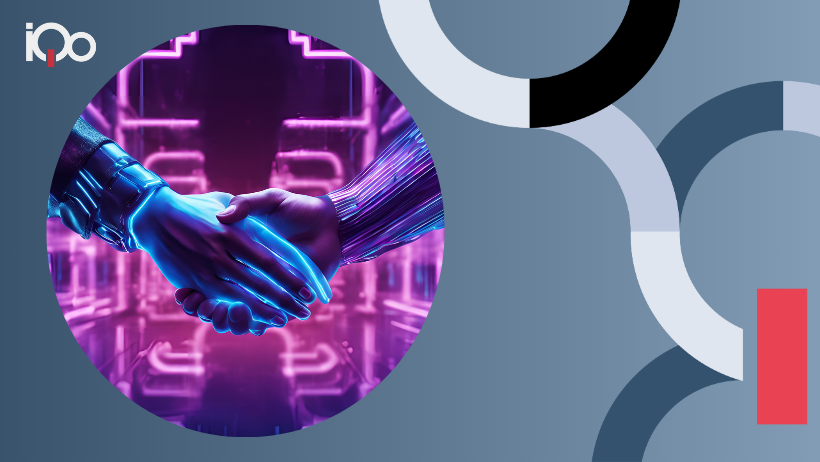
Contents
What are the risks of bias in Generative AI?
The public launch of ChatGPT on November 30, 2022 profoundly changed our relationship with Generative Artificial Intelligence (GenAI). Its use, or that of other Large Language Models (LLMs), has since become widespread. As a result, increasing number of companies are experimenting with these tools in order to support their employees in tasks as diverse as document synthesis, knowledge capitalization, email generation, customer relations, contract management and analysisHR process improvement, etc.
If this rapid rise in the power of generative AI represents an opportunity for them toaccelerate their digital transformationIt is crucial to understand the consequences of deploying generative AI solutions, and to put in place the safeguards needed to control their use.
To this end, our article examines the risks associated with GenAI's human bias.
What risks does human bias bring Generative AI?
Bias is a major risk factor for discrimination, the spread of prejudice or misinterpretation of results, and can impact AI systems at every stage of their development and deployment. The specific features of Generative AI, such as its mass adoption, ease of use, ability to interact naturally with humans, or the impression of omniscience it exudes, can exacerbate their negative impact of this bias.
1. Biases in data collection, selection and preparation
- Selection bias: conscious or unconscious selection of data that confirms pre-existing beliefs or hypotheses.
- Omission bias: systematic exclusion, whether conscious or unconscious, of certain information.
- Availability bias: tendency to use easily accessible or immediately available data, which may not be complete.
- Representativeness bias: data do not adequately reflect the diversity of people or use cases. May result from overly drastic or binary selection.
- Labeling bias: arises when labels assigned to learning data reflect subjective or stereotyped opinions, thus influencing the learning process.
2. Biases in model training
- Anchoring bias: use of an initial set of hyperparameters, for example, those used in previous work or models, which can limit exploration of configurations that might be more optimal or less biased.
- Dunning-Kruger effect: insufficient understanding of model nuances and complexities, which leads to inadequate training.
- Survivor bias: using hyperparameters that worked in previous projects or experiments, and ignoring those that didn't work but that might be relevant in the current context.
- Confirmation bias: selecting models on the basis of how well their results match our own beliefs.
3. Biases in model use
- Automation bias: overconfidence in the model's performance after deployment, overlooking the need for ongoing monitoring and evaluation.
- Illusion of knowledge: believing that the model understands subjects or answers questions more accurately than it actually does. Particularly damaging in the case of Generative AI hallucinations.
- Confirmation bias: interpreting model results in a way that confirms our pre-existing beliefs.
Our views on mastering the potential human biases found in Generative AI
Companies must anticipate the risks stemming from the inherent biases of Generative AI. Limiting these risks is crucial, and involves acting on data, algorithms and the interpretation of results.
1. Acting on Data
First of all, it is essential touse representative data sets. This implies collecting data from different sources to ensure the fair representation of all sub-populations. It is therefore important to include various demographic, geographic and socio-economic characteristics in the collection processes. Stratified random sampling is a useful technique in this respect as it ensures that all important sub-populations are adequately represented in the training data.
Exploratory data analysis is also crucial for detecting and correcting imbalances and omissions. Resampling techniques, such as oversampling minority classes or undersampling majority classes, can be applied after the fact to balance the data.
Finally, we recommend documenting data collection processes transparently, so that they critically evaluated. By involving representative teams in data collection and annotation, companies can minimize individual bias and improve the quality of the data collected.
2. Acting on algorithms
In order to limit the biases found in algorithms, it is important touse multiple evaluation metrics and to go beyond classic performance metrics to include measures of equity and justice. Indeed, critically evaluating models according to criteria that take into account the impact on different groups will help to ensure that the models are fair.
Adjusting the optimization objectives of algorithms is another important measure. By incorporating fairness constraints, techniques such as regularization can be used to avoid overfitting and reduce bias.
Finally, testing models on a variety of data sets is essential for identifying biased behavior. Debiasing techniques, such as adversarial algorithms, can be applied to neutralize biases learned during training. Weight rebalancing methods can also be used to give greater weight to under-represented classes, thus contributing to fairer models.
3. Interpreting the results
To minimize biases arising from the interpretation of results, model transparency and interpretability are crucial. Developing models that we ca exnplain allows us to understand how decisions are made, which is essential for detecting and correcting biases.
Making users aware of the potential biases of AI models and their impact on the interpretation of results is equally important, as it will teach users to adopt a critical approach when analyzing results.
Involving representative groups in the analysis and review of results will minimize confirmation bias, enabling unbiased and rigorous evaluation.
Finally, it is important to present the results in a balanced and neutral way, and to include information on their uncertainties and limitations, to enable users to make a critical and informed assessment of the conclusions provided by the modeling.
Conclusion
Limiting Generative AI biases requires a multi-dimensional, multi-actor approach. Organizing such an approach can be very difficult, which is why we recommend implementing an operational data model that will enable the principles of Data Governance to be linked with those of Usage Governance.
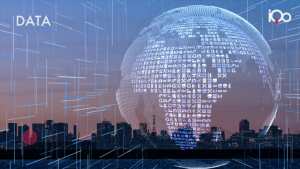
What is the environmental impact of artificial intelligence (AI)?
The rise of artificial intelligence is not without major environmental challenges. The environmental impact of AI is colossal and probably still underestimated.
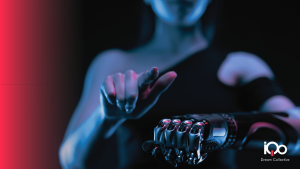
AI training: explaining and demystifying before taking the plunge
Training employees in AI is not just about responding to a current trend, it's about supporting the transformation of our businesses. All businesses are
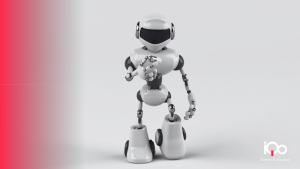
AI Readiness: is your CIO ready for Generative AI?
AI Readiness or how do you know if your company and the various business lines are ready to take advantage of the potential of AI? We have defined